While the global demand for voice recognition systems is increasing rapidly, the challenges associated with them cannot be overlooked. The blog highlights the importance of audio annotation tools/services to appropriately label audio datasets and improve the efficiency of voice recognition models. |
Speech recognition technology has become a ubiquitous part of modern life, with it finding use in a variety of applications ranging from virtual assistants like Siri and Alexa to transcription and voice-controlled devices.
According to reports from Statista, the global voice recognition market, worth $10.7 billion in 2020, is forecasted to grow to $27.16 billion by 2026.
Despite its growing popularity, speech recognition technology still faces significant challenges related to background noise, accents, and dialects, which must be overcome to improve its accuracy and reliability. Audio annotation provides a key solution to surmount these challenges, through the labeling of audio data with descriptive tags to assist in training voice recognition algorithms.
Let’s explore in detail how audio annotation can improve the efficiency of language understanding models and help in overcoming some of the top speech recognition challenges.
Applications of Automatic Speech Recognition Models
Speech recognition AI models have several use cases, ranging from on-demand audio translation to various voice assistant applications. Some of their common applications in various industries are as follows:
- Voice assistants: Speech recognition models train voice assistants, like Apple’s Siri and Amazon’s Alexa, enabling users to operate their voice-controlled devices through verbal commands.
- Dictation software: Speech recognition models transcribe spoken words into written text, facilitating the creation of emails and documents.
- Customer service: Voice recognition models in support/call centers allow customers to interact with automated systems using voice commands.
- Education: Speech recognition models can be used to provide feedback to students on their pronunciation and speaking skills.
- Healthcare: Speech recognition models can transcribe doctors’ notes and allow patients to interact with their electronic health records using voice commands.
- Transportation: Speech recognition technology is used in self-driving vehicles, allowing passengers to give voice instructions to the vehicle.
- Home automation: Speech recognition technology is used in smart home systems to let users control their appliances and devices via voice commands.
Top Speech Recognition Challenges & How to Solve Them with Audio Annotation
Various voice recognition challenges affect the efficiency of automatic speech recognition (ASR) models. Some of the common ones are:
- Background Noise
Ambient noise, including echo, cross-talk, and white noise, can significantly reduce the accuracy of ASR models by introducing sounds that are not part of the spoken words.
Reports according to Statista indicate that accuracy is the leading barrier to global voice technology adoption. The accuracy and efficiency of speech recognition models are measured by their Word Error Rate (WER). A lower WER indicates a more accurate and efficient ASR model.
Unfortunately, background noise can increase the WER for voice recognition models and make it challenging for them to distinguish between similar-sounding words or phrases, resulting in further inaccuracies in the transcribed text.
Solution:
Audio annotation can help in identifying and tagging specific sounds (such as echo, cross-talk, etc.) for various audio files. These tags will allow ASR models to filter unwanted background noise for better accuracy and efficiency. Additionally, labeling the dataset with annotations can help the ASR model recognize the difference between words or phrases that sound similar, which can help reduce the number of errors (WER) in transcription.
- Overlapping Speech
When multiple speakers speak at the same time, their speeches overlap, making it difficult for voice recognition models to distinguish between their phrases and intent. This can lead to high WER, inaccuracies, and inefficiency of ASR models.
Solution:
Through audio annotation, we can help train the ASR model to recognize and transcribe each speaker’s words separately, even in multi-speaker conversations. By adding annotations to the dataset, the ASR model can differentiate better between similar-sounding words spoken by different speakers. This enables the model to identify the speaker and transcribe their phrases separately, resulting in a more accurate transcription of overlapping speech.
- Differences in Linguistic Expressions
Variation in speech patterns such as different accents, dialects, and languages, makes it challenging for voice recognition models to accurately identify and transcribe spoken words. Various factors such as age, gender, and context contribute to the deviations in linguistic expression.
Also, if a voice recognition model is trained on a language, it may not recognize different accents or dialects in the same language. This can be particularly problematic for people with non-standard accents or dialects, as they may not be adequately represented in the training data used to develop the voice recognition model.
Solution:
Train the ASR model on various voice samples to improve its ability to recognize various accents and speeches accurately. Also, through audio annotation, you can label metadata such as speaker identity, location, and contextual information, which can help voice recognition models better understand the variations in speech patterns.
- Non-standard Vocabulary
ASR models may find it challenging to recognize and understand specialized terms, slang, and non-standard vocabulary if they are not trained on specifically labeled datasets. This limitation can result in inaccurate transcription and reduced overall performance, as the models may misinterpret or fail to recognize certain words or phrases.
Solution:
Audio annotation can overcome this challenge by providing the models with labeled audio data that includes a wide range of non-standard vocabulary, including slang and colloquial terms. This labeled training dataset will help voice recognition models learn to recognize and transcribe these terms accurately for better efficiency and accuracy.
- Variations in Emotional and Tonal Expressions
Detecting and interpreting users’ context and intent can be challenging for ASR models due to the variations in emotional and tonal expression. ASR models may find it difficult to distinguish between similar-sounding words or phrases delivered in different emotional or tonal contexts, leading to inaccurate transcriptions and reduced performance.
Solution:
Through speech annotation, we can provide additional contextual information about the emotional and tonal expressions present in the audio data. We can label the audio with metadata about the speaker’s emotional state, tone, and other relevant contextual details. By incorporating this information into the model’s training data, voice recognition models can learn to differentiate between subtle variations in emotional and tonal expression for improved accuracy and performance.
- Data Biases
Biases can occur for the voice recognition system if the training dataset consists of input only from speakers of a particular region. Such ASR models can perform poorly when interpreting input from individuals with different accents or speech patterns.
One of Stanford’s research showed that the word error rate for speakers of African descent was nearly double that of speakers of American descent after analyzing the thousands of audio snippets by leading voice-to-text service providers like Amazon, Microsoft, Google, IBM, etc. The primary reason for the model’s lack of accuracy was the presence of data biases.
Solution:
To address the issue of biased data, it is important to collaborate with a wide range of users to ensure that voice recognition technology is developed in an inclusive manner that considers all users’ needs.
How Can Audio Annotation Enhance the Accuracy of Speech Recognition Models?
Speech annotation assigns descriptive labels or tags to audio data to improve the training and efficiency of natural language and voice recognition models. It involves listening to audio recordings, identifying specific sounds, speech, music, etc., and adding meaningful metadata to improve ASR models’ efficiency.
Here are some prominent benefits of incorporating data annotation in voice recognition models:
- Improves precision of ASR models: The more accurately the speech data is labeled with critical information, the higher the precision of the voice recognition system.
- Enhances user experience: When the speech data is labeled with the appropriate information, the ASR-based applications or voice assistants can provide relevant assistance to improve the user experience.
- Builds users’ trust: The incorporation of annotated speech data to include a diverse range of voices can make voice recognition models more inclusive and representative of different demographics, ultimately building trust with users who may have felt excluded or marginalized by the technology.
You can leverage data annotation to improve the performance and efficiency of voice recognition models in the following two ways:
- Hire a team of experienced data annotation professionals who can efficiently collect and categorize audio data from diverse sources, followed by annotating it with critical information based on their relevant linguistic expertise.
- Use automated audio annotation tools like Labelbox, Audacity, Annotate Audio, etc., to identify and label certain types of speech or sounds, followed by implementation of a human-in-the-loop approach for verifying and correcting the labels.
Conclusion
The importance of correctly labeled speech data cannot be overstated in today’s world, where voice recognition technology is increasingly prevalent. The speech recognition challenges posed by data biases and complex data can be overcome with audio annotation. By leveraging reliable audio data annotation services or tools, you can swiftly and efficiently create more accurately labeled training datasets for improved performance of voice recognition models.
Also Read: Unveiling the Iconic Seattle Kraken Logo and How to Download it for Free in Vector File Format
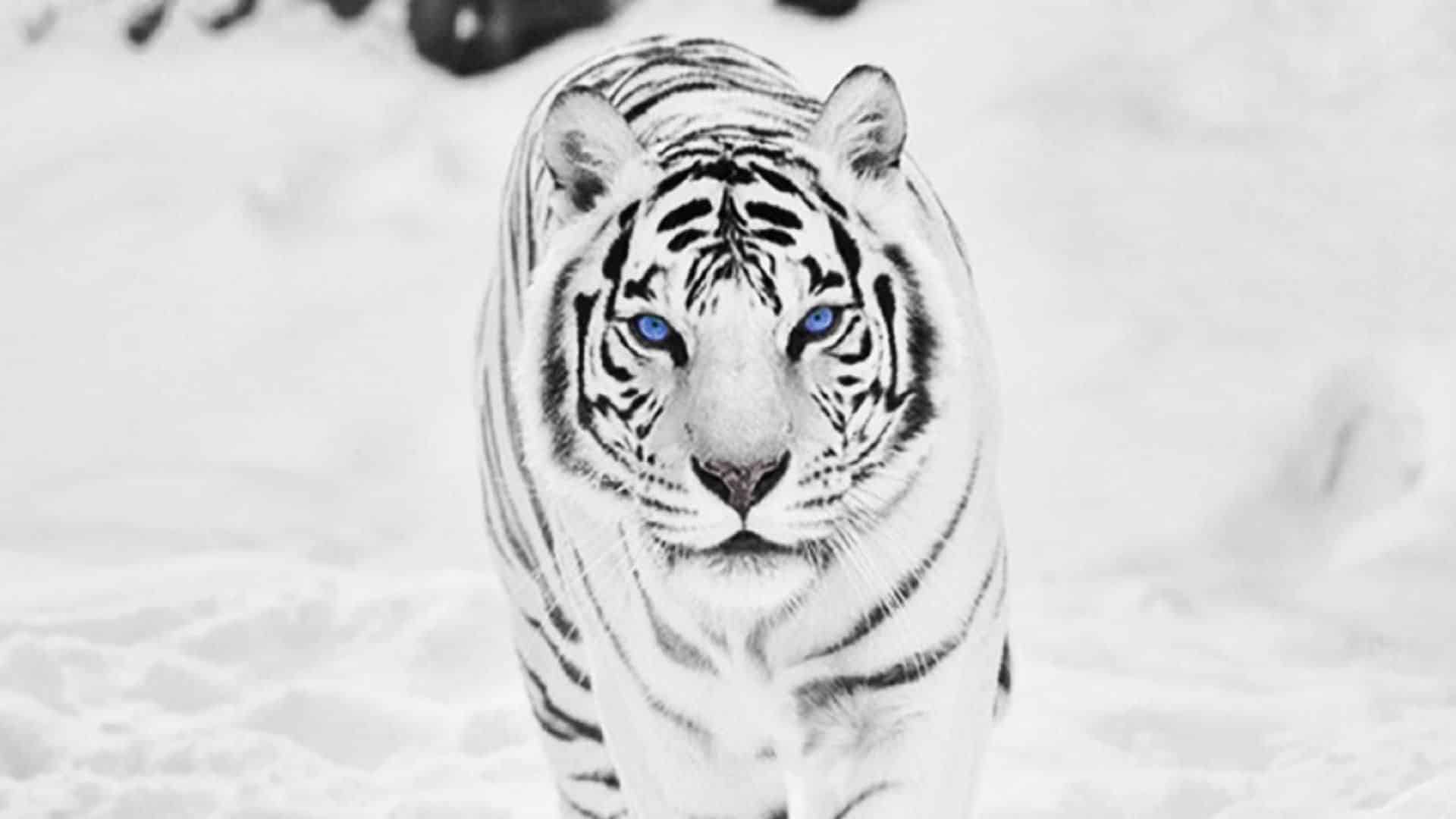
Crafting words to inspire, engage and motivate. 10+ years of content writing, SEO, digital marketing and blogging experience. Ready to help your brand reach its potential!